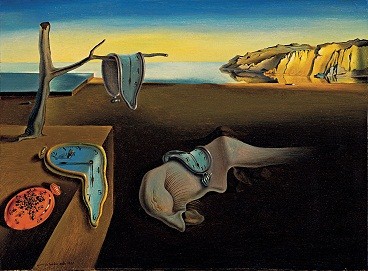
Salvador Dali, The Persistence of Memory, 1931
Our new paper, led by Warrick Roseboom, is out now (open access) in Nature Communications. It’s about time.
More than two thousand years ago, though who knows how long exactly, Saint Augustine complained “What then is time? If no-one asks me, I know; if I wish to explain to one who asks, I know not.”
The nature of time is endlessly mysterious, in philosophy, in physics, and also in neuroscience. We experience the flow of time, we perceive events as being ordered in time and as having particular durations, yet there are no time sensors in the brain. The eye has rod and cone cells to detect light, the ear has hair cells to detect sound, but there are no dedicated ‘time receptors’ to be found anywhere. How, then, does the brain create the subjective sense of time passing?
Most neuroscientific models of time perception rely on some kind internal timekeeper or pacemaker, a putative ‘clock in the head’ against which the flow of events can be measured. But despite considerable research, clear evidence for these neuronal pacemakers has been rather lacking, especially when it comes to psychologically relevant timescales of a few seconds to minutes.
An alternative view, and one with substantial psychological pedigree, is that time perception is driven by changes in other perceptual modalities. These modalities include vision and hearing, and possibly also internal modalities like interoception (the sense of the body ‘from within’). This is the view we set out to test in this new study, initiated by Warrick Roseboom here at the Sackler Centre, and Dave Bhowmik at Imperial College London, as part of the recently finished EU H2020 project TIMESTORM.
*
Their idea was that one specific aspect of time perception – duration estimation – is based on the rate of accumulation of salient events in other perceptual modalities. More salient changes, longer estimated durations. Fewer salient changes, shorter durations. He set out to test this idea using a neural network model of visual object classification modified to generate estimates of salient changes when exposed to natural videos of varying lengths (Figure 1).

Figure 1. Experiment design. Both human volunteers (a, with eye tracking) and a pretrained object classification neural network (b) view a series of natural videos of different lengths (c), recorded in different environments (d). Activity in the classification networks is analysed for frame-to-frame ‘salient changes’ and records of salient changes are used to train estimates of duration – based on the physical duration of the video. These estimates are then compared with human reports. We also compare networks trained on gaze-constrained video input versus ‘full frame’ video input.
We first collected several hundred videos of five different environments and chopped them into varying lengths from 1 sec to ~1 min. The environments were quiet office scenes, café scenes, busy city scenes, outdoor countryside scenes, and scenes from the campus of Sussex University. We then showed the videos to some human participants, who rated their apparent durations. We also collected eye tracking data while they viewed the videos. All in all we obtained over 4,000 duration ratings.
The behavioural data showed that people could do the task, and that – as expected – they underestimated long durations and overestimated short durations (Figure 2a). This ‘regression to the mean’ effect is known as Vierodt’s law in the time perception literature and is very well known. Our human volunteers also showed biases according to the video content, rating busy (e.g., city) scenes as lasting longer than non-busy (e.g., office) scenes of the same physical duration. This is just as expected, if duration estimation is based on accumulation of salient perceptual changes.
For the computational part, we used AlexNet, a pretrained deep convolutional neural network (DCNN) which has excellent object classification performance across 1,000 classes of object. We exposed AlexNet to each video, frame by frame. For each frame we examined activity in four separate layers of the network and compared it to the activity elicited by the previous frame. If the difference exceeded an adaptive threshold, we counted a ‘salient event’ and accumulated a unit of subjective time at that level. Finally, we used a simple machine learning tool (a support vector machine) to convert the record of salient events into an estimate of duration in seconds, in order to compare the model with human reports. There are two important things to note here. The first is that the system was trained on the physical duration of the videos, not on the human estimates (apparent durations). The second is that there is no reliance on any internal clock or pacemaker at all (the frame rate is arbitrary – changing it doesn’t make any difference).
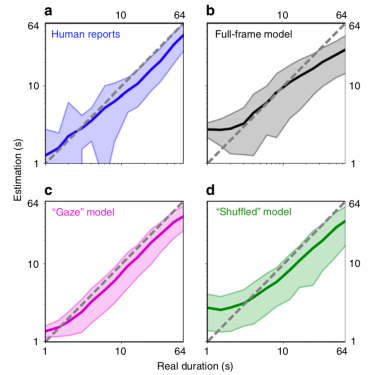
Fig 2. Main results. Human volunteers can do the task and show characteristic biases (a). When the model is trained on ‘full-frame’ data it can also do the task, but the biases are even more severe (b). There is a much closer match to human data when the model input is constrained by human gaze data (c), but not when the gaze locations are drawn from different trials (d).
There were two key tests of the model. Was it able to perform the task? More importantly, did it reveal the same pattern of biases as shown by humans?
Figure 2(b) shows that the model indeed performed the task, classifying longer videos as longer than shorter videos. It also showed the same pattern of biases, though these were more exaggerated than for the human data (a). But – critically – when we constrained the video input to the model by where humans were looking, the match to human performance was incredibly close (c). (Importantly, this match went away if we used gaze locations from a different video, d). We also found that the model displayed a similar pattern of biases by content, rating busy scenes as lasting longer than non-busy scenes – just as our human volunteers did. Additional control experiments, described in the paper, rule out that these close matches could be achieved just by changes within the video image itself, or by other trivial dependencies (e.g., on frame rate, or on the support vector regression step).
Altogether, these data show that our clock-free model of time-perception, based on the dynamics of perceptual classification, provides a sufficient basis for capturing subjective duration estimation of visual scenes – scenes that vary in their content as well as in their duration. Our model works on a fully end-to-end basis, going all the way from natural video stimuli to duration estimation in seconds.
*
We think this work is important because it comprehensively illustrates an empirically adequate alternative to ‘pacemaker’ models of time perception.
Pacemaker models are undoubtedly intuitive and influential, but they raise the spectre of what Daniel Dennett has called the ‘fallacy of double transduction’. This is false idea that perceptual systems somehow need to re-instantiate a perceived property inside the head, in order for perception to work. Thus perceived redness might require something red-in-the-head, and perceived music might need a little band-in-the-head, together with a complicated system of intracranial microphones. Naturally no-one would explicitly sign up to this kind of theory, but it sometimes creeps in unannounced to theories that rely too heavily on representations of one kind or another. And it seems that proposing a ‘clock in the head’ for time perception provides a prime example of an implicit double transduction. Our model neatly avoids the fallacy, and as we say in our Conclusion:
“That our system produces human-like time estimates based on only natural video inputs, without any appeal to a pacemaker or clock-like mechanism, represents a substantial advance in building artificial systems with human-like temporal cognition, and presents a fresh opportunity to understand human perception and experience of time.” (p.7).
We’re now extending this line of work by obtaining neuroimaging (fMRI) data during the same task, so that we can compare the computational model activity against brain activity in human observers (with Maxine Sherman). We’ve also recorded a whole array of physiological signatures – such as heart-rate and eye-blink data – to see whether we can find any reliable physiological influences on duration estimation in this task. We can’t – and the preprint, with Marta Suarez-Pinilla – is here.
*
Major credit for this study to Warrick Roseboom who led the whole thing, with the able assistance of Zaferious Fountas and Kyriacos Nikiforou with the modelling. Major credit also to David Bhowmik who was heavily involved in the conception and early stages of the project, and also to Murray Shanahan who provided very helpful oversight. Thanks also to the EU H2020 TIMESTORM project which supported this project from start to finish. As always, I’d also like to thank the Dr. Mortimer and Theresa Sackler Foundation, and the Canadian Institute for Advanced Research, Azrieli Programme in Brain, Mind, and Consciousness, for their support.
*
Roseboom, W., Fountas, Z., Nikiforou, K., Bhowmik, D., Shanahan, M.P., and Seth, A.K. (2019). Activity in perceptual classification networks as a basis for human subjective time perception. Nature Communications. 10:269.